Identifying the root cause of cable network problems with machine learning
Mar 14, 2022·
,,,,·
0 min read

Georg Heiler
Thassilo Gadermaier
Thomas Haider
Allan Hanbury
Peter Filzmoser
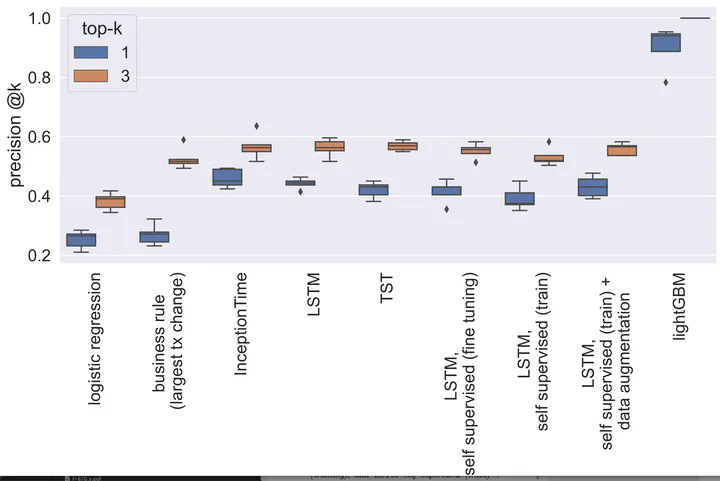
Abstract
Good quality network connectivity is ever more important. For hybrid fiber coaxial (HFC) networks, searching for upstream high noise in the past was cumbersome and time-consuming. Even with machine learning due to the heterogeneity of the network and its topological structure, the task remains challenging. We present the automation of a simple business rule (largest change of a specific value) and compare its performance with state-of-the-art machine-learning methods and conclude that the precision@1 can be improved by 2.3 times. As it is best when a fault does not occur in the first place, we secondly evaluate multiple approaches to forecast network faults, which would allow performing predictive maintenance on the network.
Type
Publication
Preprint: Identifying the root cause of cable network problems with machine learning